To understand the future of AI, take a look at the failings of Google Translate
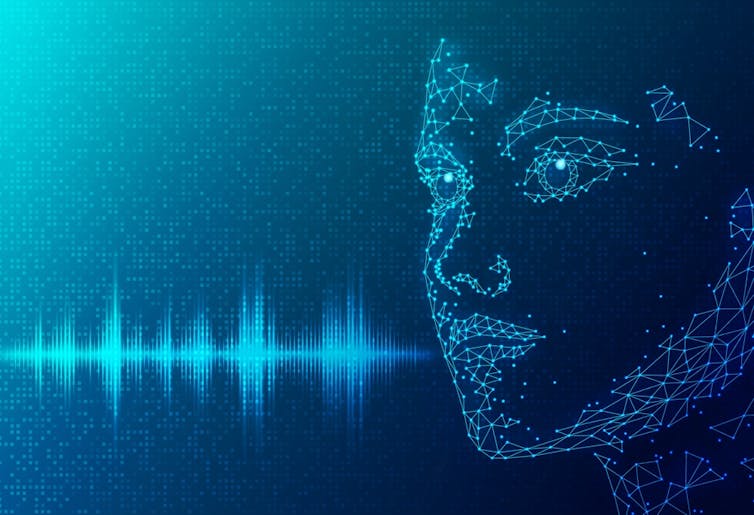
To understand the future of AI That’s a pretty compelling statement—and you’re not wrong. The trajectory of AI development can often be seen in the successes and the shortcomings of early tools like Google Translate. Want to dive into some key failings that reveal deeper insights into where AI might be headed?
Here are a few that stand out:
1. Lack of Deep Contextual Understanding
To understand the future of AI Google Translate can turn “I’m feeling blue” into a literal color statement in another language. That’s because it often misses idioms, double meanings, and emotional nuance. It processes words and phrases, not the context or intent behind them.
Future Insight: The next generation of AI must move beyond pattern recognition and toward a deeper, semantic grasp of language. It needs to understand not just how words relate to each other, but why people say them in the first place.
2. Bias and Cultural Blind Spots
Translate “doctor” from English into some gendered languages and it might default to the male form. Translate “nurse” and it might default to female. To understand the future of AI These biases aren’t built intentionally—they emerge from the training data. AI reflects the world we feed into it, and sometimes that world is unfair.
Future Insight: To understand the future of AI systems must be built with safeguards that actively mitigate bias. This includes more diverse datasets, transparent algorithms, and feedback loops that include human ethics.
3. Overreliance on Massive Data
Google Translate works well for widely spoken languages, but for less-resourced ones? Not so much. It’s a classic case of “rich get richer”—the more data a language has, the better the results.
Future Insight: Tomorrow’s AI may need to do more with less. That could mean smaller, more adaptable models or training techniques that rely on linguistic universals and cross-lingual learning rather than brute-force data.
4. No Real-World Experience
Google Translate works well for widely spoken languages, but for less-resourced ones? Not so much. It’s a classic case of “rich get richer”—the more data a language has, the better the results.
Future Insight: Tomorrow’s AI may need to do more with less. That could mean smaller, more adaptable models or training techniques that rely on linguistic universals and cross-lingual learning rather than brute-force data.

Conclusion: A Mirror and a Map
The limitations of Google Translate show us two things.To understand the future of AI First, they mirror the limitations of today’s mainstream AI: smart but superficial, powerful but rigid. Second, they map out what needs to change—contextual understanding, ethical design, efficient learning, and interactive intelligence.
If you want to see the future of AI, don’t just look at what’s working. Look at what’s failing—and ask why.
he computer scientists Rich Sutton and Andrew Barto have been recognised for a long track record of influential ideas with this year’s Turing Award, the most prestigious in the field. Sutton’s 2019 essay The Bitter Lesson, for instance, underpins much of today’s feverishness around artificial intelligence (AI).
He argues that methods to improve AI that rely on heavy-duty computation rather than human knowledge are “ultimately the most effective, and by a large margin”. To understand the future of AI This is an idea whose truth has been demonstrated many times in AI history. Yet there’s another important lesson in that history from some 20 years ago that we ought to heed.
Today’s AI chatbots are built on large language models (LLMs), which are trained on huge amounts of data that enable a machine to “reason” by predicting the next word in a sentence using probabilities.
Useful probabilistic language models were formalised by the American polymath Claude Shannon in 1948, citing precedents from the 1910s and 1920s. Language models of this form were then popularised in the 1970s and 1980s for use by computers in translation and speech recognition, in which spoken words are converted into text.
The first language model on the scale of contemporary LLMs was published in 2007 and was a component of Google Translate, which had been launched a year earlier. Trained on trillions of words using over a thousand computers, it is the unmistakeable forebear of today’s LLMs, even though it was technically different.
It relied on probabilities computed from word counts, whereas today’s LLMs are based on what is known as transformers. First developed in 2017 – also originally for translation – these are artificial neural networks that make it possible for machines to better exploit the context of each word.
The pros and cons of Google Translate
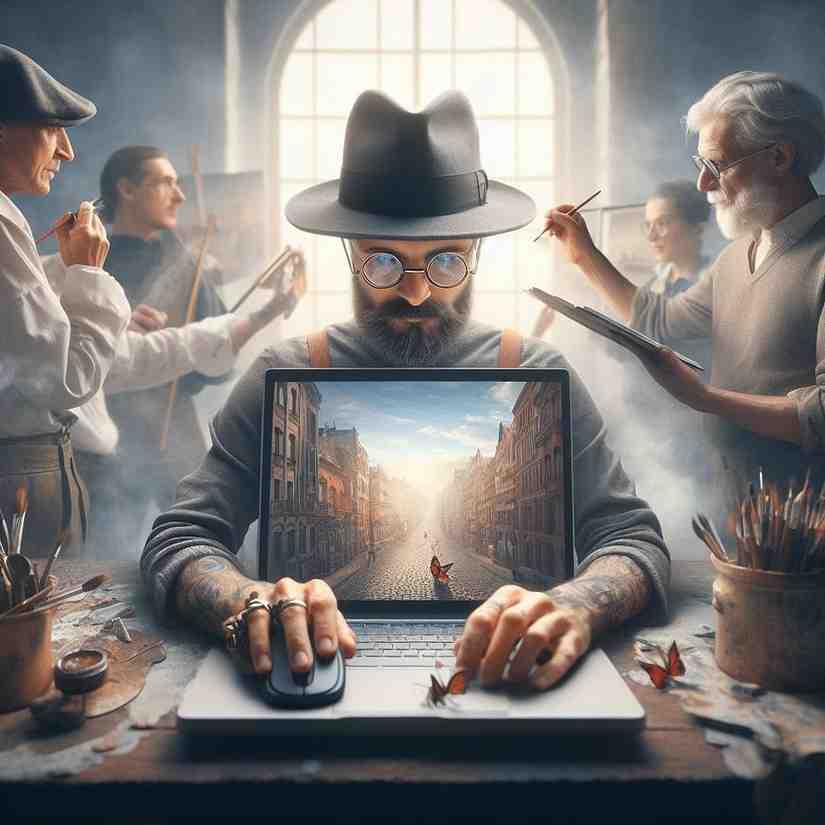
Machine translation (MT) has improved relentlessly in the past two decades, driven not only by tech advances but also the size and diversity of training data sets. Whereas Google Translate started by offering translations between just three languages in 2006 – English, Chinese and Arabic – today it supports 249. Yet while this may sound impressive, it’s still actually less than 4% of the world’s estimated 7,000 languages.
Between a handful of those languages, like English and Spanish, translations are often flawless. Yet even in these languages, the translator sometimes fails on idioms, place names, legal and technical terms, and various other nuances.
Between many other languages, the service can help you to get the gist of a text, but often contains serious errors. The largest annual evaluation of machine translation systems – which now includes translations done by LLMs that rival those of purpose-built translation systems – bluntly concluded in 2024 that “MT is not solved yet”.
Machine translation is widely used in spite of these shortcomings: as far back as 2021, the Google Translate app reached 1 billion installs. Yet users still appear to understand that they should use such services cautiously: a 2022 survey of 1,200 people found that they mostly used machine translation in low-stakes settings, like understanding online content outside of work or study. Only about 2% of respondents’ translations involved higher stakes settings, including interacting with healthcare workers or police.
Sure enough, there are high risks associated with using machine translations in these settings. Studies have shown that machine-translation errors in healthcare can potentially cause serious harm, and there are reports that it has harmed credible asylum cases. It doesn’t help that users tend to trust machine translations that are easy to understand, even when they are misleading.
Knowing the risks, the translation industry overwhelmingly relies on human translators in high-stakes settings like international law and commerce. Yet these workers’ marketability has been diminished by the fact that the machines can now do much of their work, leaving them to focus more on assuring quality.
Many human translators are freelancers in a marketplace mediated by platforms with machine-translation capabilities. It’s frustrating to be reduced to wrangling inaccurate output, not to mention the precarity and loneliness endemic to platform work. Translators also have to contend with the real or perceived threat that their machine rivals will eventually replace them – researchers refer to this as automation anxiety.
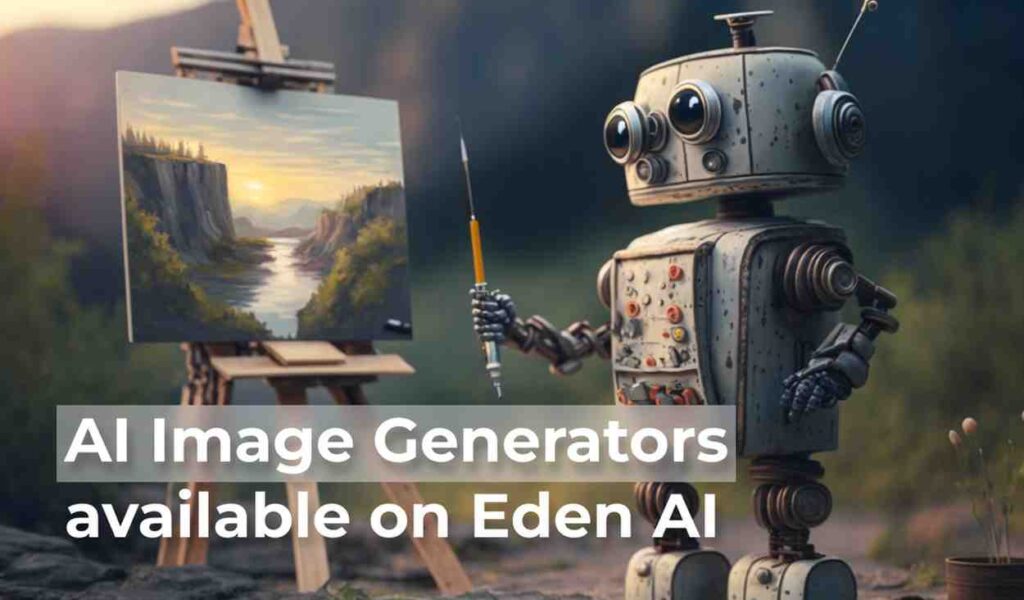